Is predictive modeling the key to finding and removing lead pipes?
By October 2024, all water utilities in the U.S. will have to submit a service line inventory to the EPA. Confirming service line materials throughout an entire system requires field verification programs, which take time and resources to complete. Katie Deheer from Trinnex sat down to explain how utilities can save time and money with predictive modeling tools.
Q: What can predictive modeling help utilities do?
Predictive modeling can be an all-encompassing term that covers at least one aspect of what we do, which is machine learning. Machine learning involves using known data that is preferably high-quality to make predictions about unknown service lines.
Let's say you know a portion of your materials through physical verification, and you look at the trends and patterns associated with that information and predict unknown data. That's essentially what predictive modeling is, using what we know to try and ascertain information about what we don't know.
Q: What makes a utility a good candidate to explore the option of predictive modeling?
Any utility that has a significant number of unknowns in their service line inventory can take advantage of predictive modeling — that means that there’s a pretty good pool of utilities out there that can take advantage of this technology!
And we have to consider data quality, right? So the data going in is going to be critical in determining the quality of the output that you get. If a utility is not confident that they have good data to create a predictive model, we can help them collect that data, validate it, and make sure that we are putting good data into our model.
Q: How do you train these models and what data are you using to do it?
We start with a representative subset of field verified service line materials. It’s important that the subset is representative of the unknowns in order to have a reliable model. The field verifications need to be unbiased and representative of the unknowns in your service area.
Then we take those field verifications and we add other information such as service line characteristics, assessor data and demographic data. We use anything we can get our hands on to ascertain trends and patterns around service line material in that set of known data and use that information to predict for the unknowns what the material may be.
Q: Is there current regulatory guidance in place for the use of predictive modeling?
The EPA recognizes predictive modeling as a valuable tool for prioritizing field verifications and helping to guide the development of service line inventories. This leaves the acceptance of predictive modeling as a verification method up to the individual states.
Trinnex and CDM Smith are in discussions with the EPA and state regulatory agencies across the country to share best practices and provide education around predictive modeling, so if you're not sure whether your state will accept it, check out our map.
Predictive modeling is an iterative process that can save utilities time and money completing field verifications.
Q: Are you able to coordinate with utilities and state regulators to get approval for utilities to use predictive modeling?
Absolutely. We start out by describing our approach formally to the state regulator and answering their questions. If they have specific guidance or things they’re looking for, we tailor our message to make sure that we address those concerns of that particular state. We have also seen that states and water utilities appreciate when we bring in our experience working with other states because it helps to support the community of learning.
Q: Tell me about the concept of responsible AI. Why is it important and how does leadCAST Predict fall under the responsible AI umbrella?
With leadCAST Predict, we bake responsible AI into everything we do. Responsible AI is all about governing the use of AI to ensure it's fair, reliable and accurate. When it comes to this concept, there’s a lot of pillars underneath around transparency, communication, expectation setting, data quality and representation.
When it comes to representation, we start our process with a representative sample to ensure there’s no hidden biases in the sample or subset of field verification that we’re using to train the model. We try to make it as representative as possible of the population that we’re modeling for, which in this case is the unknowns in the water system.
The other aspect is data quality. For predictive modeling, you have probably heard the term ‘garbage in, garbage out.’ But it’s more nuanced than that. Data going into predictive models and the outcome of the model need to be scrutinized by experts who really understand the water system.
Learn how leadCAST Predict can save you time and money in the inventory process by reaching out to me.
Q: Where have you seen this technology improve the lead service line replacement process?
I'll give you an example of a community that we're working with in New Jersey. They have very little lead or galvanized requiring replacement in their system that they know of, but they have a pretty aggressive plan to replace all of it in a pretty aggressive time frame. They need to focus more on which properties they can deprioritize, right? We don't want to spend our time and resources going to properties that have a really low probability of having a lead service line.
Our team built a predictive model for them and it's an iterative process. In the first few iterations, the model has been able to classify just under half of their unknowns as having less than 10% probability of being lead, with greater than 95% accuracy.
They can also see that there’s a big chunk of properties that they should not focus on, enabling them to direct time and resources to higher priorities and target the specific properties that are more likely to have lead service lines. It's twofold approach.
Q: We've heard you’re the best at convincing skeptical regulators this method is the most cost efficient and more effective at finding these lead service lines. What do you say to give regulators confidence in these predictions?
The important thing to remember is that it’s not ‘all or nothing’. There’s not a situation where we run the model once, predict the material with a few 100 records, and then put that material in the inventory and don't re-visit it. It’s a very iterative process! First, we start out with a representative sample, and we iterate through the process and then field test.
The model gives you predictions to verify — if you find a large gap between what you're verifying and what the model predicted right off the bat, then there’s likely a problem, so we will readjust and repeat that cycle many times. You get to see it evolve over time, demonstrate the model’s efficacy in the field, document it, and report it to regulators.
This concept of it not being an ‘all or nothing’ approach allows us to look at it more granularly. The model gives a range of probabilities from 0-100%, and we don’t just draw a line down the middle and decide that anything less than 50% is going to be called non-lead. Utilities also have less funding to go out and inspect every single service line. It’s millions and millions of dollars, and no utility can feasibly do that. We can show this information to regulators and help them understand and have more confidence in these predictions.
Katie Deheer has over a decade of experience implementing the latest technology solutions for her clients. Her work in data analytics spans across industries, from engineering and construction, to telecom, to commercial banking, and more. She currently leads the design, development and deployment of insightful digital solutions at Trinnex.
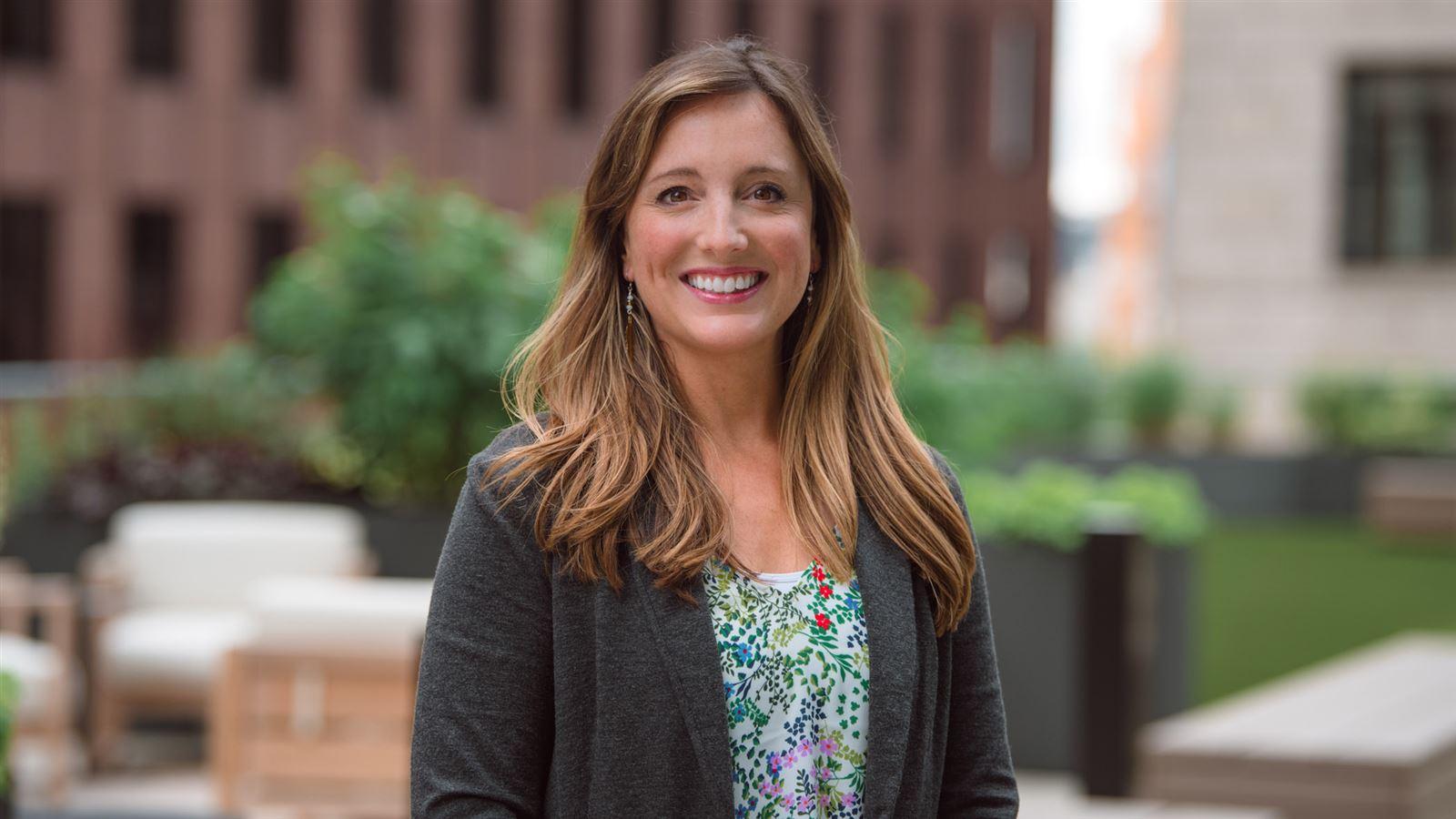
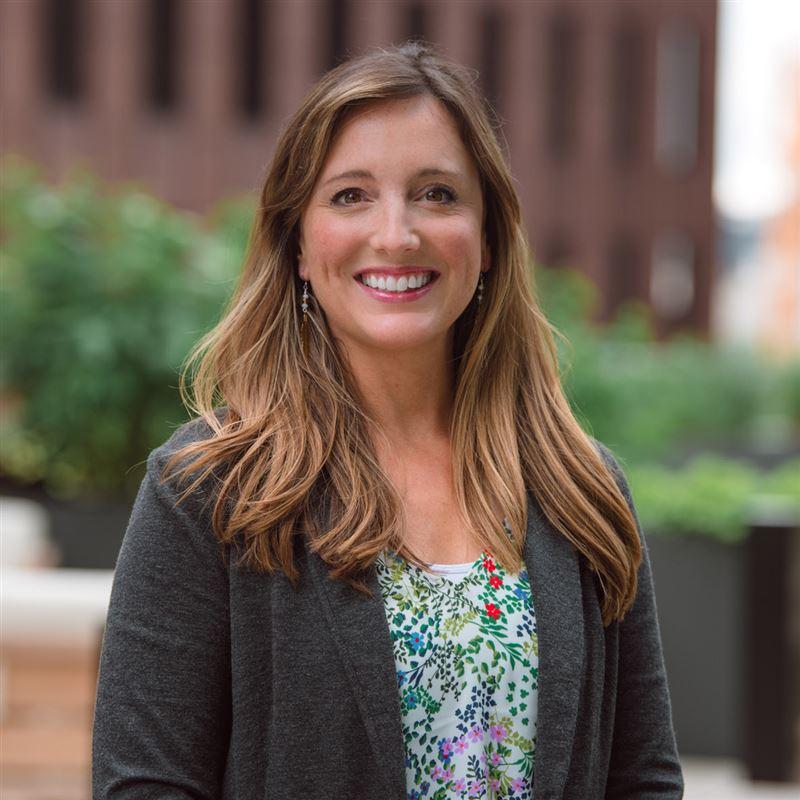
Any utility that has a significant number of unknowns in their service line inventory can take advantage of predictive modeling.