FAQs about Using Machine Learning or Predictive Modeling for Inventory Development
Will machine learning/predictive modeling be approved by the state regulators for use in inventory development? Or do I need to dig up every unknown service line in my system?
This is a question we get often because it has a tremendous impact on the time and cost associated with developing a service line inventory, especially when a system has many unknowns. Although the EPA has not provided clear guidance and most states have not made a decision on whether or not to accept a form of predictive modeling, some guidance is starting to surface indicating that predictive modeling will likely be an acceptable method for inventory development under certain conditions. We review below why we think it will be accepted, under what conditions, and the current guidance that is available.
Why do we believe predictive modeling/machine learning will be an acceptable method for inventory development?
It has been generally accepted by state regulators that defined characteristics of a water service line can be used to determine that a pipe is not lead, such as year of installation on the utility side and home construction date both beyond a certain year, or diameter being above a certain size believed to not be of lead material. It is also generally accepted to consider historic records such as tap cards, standard specs or plumbing codes as a source.
Predictive models help to validate the assumptions made and the accuracy of those records and expand on the assumptions with either random or targeted inspections and then reevaluation of the assumptions. This process is repeated until a desired accuracy or confidence level is achieved.
Machine learning is a method that can determine the accuracy through methods such as cross validation and is beneficial in evaluating the assumptions by quantifying the importance of data attributes through feature selection. It can also help improve on the assumptions by identifying patterns that are not as apparent to further increase the accuracy. For example, including location data in the model may result in a pattern of where lead was used / not used by a specific contractor performing installations many decades ago. Below are examples using Trinnex's lead management system, leadCAST.
What do we think the requirements will be for the predictions to be accepted?
Although other states and the EPA have not put out official guidance, most regulators that we speak with say that they do not expect the utilities to physically inspect every single line. Using predictive modeling or machine learning to confirm assumptions and records will improve the accuracy of inventories. We expect the requirements to include a minimum confidence level in the model results and enough physical verifications for the regulators to feel comfortable that all assumptions have been appropriately validated.
It is also important to always have a standard operating procedure (SOP) in place to document physical verifications in the future to continue to validate the model and make changes where necessary.
What guidance is currently available for using predictive modeling/machine learning?
Michigan’s Department of Environment, Great Lakes and Energy (EGLE) has provided guidelines for using predictive tools to determine the materials of unknown service lines based on physical verifications of a random sampling.
EGLE requires using a representative, uniformly random number of service lines to be verified based on:
- Utilities with fewer than 1,500 unknown service lines must physically verify at least 20 percent of the total number of unknowns.
- Utilities with more than 1,500 unknowns must physically verify enough lines to reach a 95 percent confidence level.
The physical verifications of the unknowns require three or four points of verification – the interior, the exterior of the customer side of the line, the exterior of the utility side of the line and sometimes the connection to the main (unless a utility assumes galvanized to always have a lead gooseneck and can provide proof that lead goosenecks were not used with any other materials). The results are evaluated and used to predict the remaining unknowns in the system. As additional verifications are performed, the assumptions are updated and the model continuously improves.
This method was presented on an Association of State Drinking Water Administrators (ASDWA) sponsored webinar available at: https://www.asdwa.org/event/lead-service-line-inventory-symposium/.
This section will be updated as more guidance is provided by the EPA, the states or other organizations.
Should I go ahead and use machine learning or could I be wasting my money?
We believe it is important to get started on the inventory right away. Machine learning can be used to organize data and target locations for inspections even if it is not ultimately approved as a final verification method. Even if a state requires every home to be physically verified, machine learning will help to prioritize inspections for what you want to verify first – the likely not lead service lines that you can check off the unknown list or the likely lead lines that you want to go ahead and replace before October 2024.
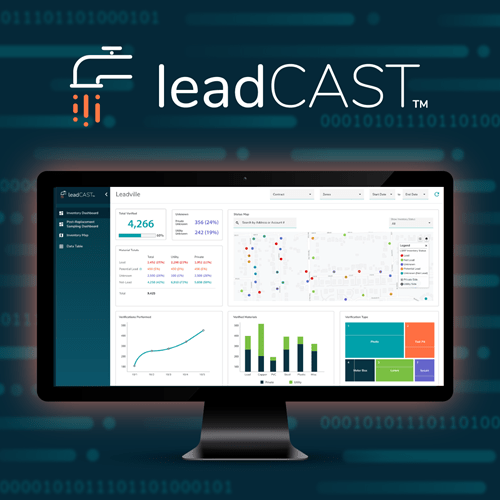
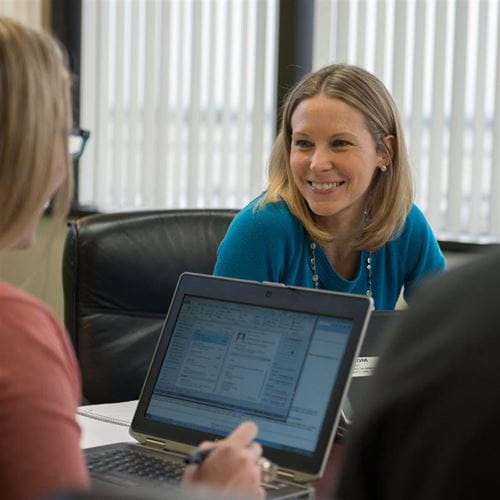