Drones, Remote Sensing, and Machine Learning to Evaluate Environmental Projects
Our recent webinar, Drones, Remote Sensing, and Machine Learning to Evaluate Environmental Projects presented by Brendan Brown and Andrew Reicks, discusses how high-resolution sensors and machine learning models can be used to help land managers and stakeholders make decisions for their environmental restoration projects. Here is an overview of what they covered:
Instruments
There are many different drone-mountable sensors that are applicable to environmental projects. The two that are focused on in this webinar are:
-
Digital Cameras
-
These can be used to produce:
-
Topographic maps
-
3D site models
-
Cross sections
-
Videos
-
Photos
-
Digital Cameras typically provide resolutions of one inch per pixel
-
Multispectral Sensors
-
These can be used to provide:
-
Pervious versus impervious surface assessments
-
Vegetation monitoring through spectral signatures of land cover, as different plant species have different spectral signatures
-
Color infrared imaging (near infrared: chlorophyll reflects, and water absorbs, which can reveal vegetation cover and health)
Exploring the Machine Learning Methods
Drone flight preparation includes multiple facets, such as setting up the ground control points (GCPs) and establishing the landing zone and benchmarks. From aerial images, you can derive multiple data sets; one method of data processing is structure from motion (SfM), in which 3D models can be made from overlapping 2D images. Ground truthing and ground control points are crucial to ensuring model accuracy.
Usually with data collection for a site, the goal is to either classify something (determine the “what”) or quantify something (determine how much). Machine learning and AI is used to do this for large data sets. However, not every software package is a best fit for answering certain questions pertaining to a site, which is where custom developed analysis comes into play. Custom developed analysis will be discussed in the following case studies.
Case Studies
Tidal Salt Marsh Restoration
The first case discussed was the Tidal Salt Marsh Restoration project monitored by CDM Smith, in which an old bridge needed to be replaced, and the adjacent tidal marsh was restored and replanted. CDM Smith collected large, drone-derived multispectral datasets and applied machine learning to analyze restoration trajectories. The team assessed multiple environmental parameters including topography, vegetative cover, vegetative height, inundated areas, soil properties, and the effects of climate change on this site. Using the drone data, CDM Smith developed a high-resolution, high accuracy 3D site model of vegetation and land surface. Under the right conditions, drones can be used to get near survey-grade elevations, and this drone data allowed CDM Smith to provide the client with the locations where the contractor did not meet the target elevations for grading. Additionally, cross sections can be generated, and volumes can also be calculated from digital elevation models (DEMs).
For this restoration project, multispectral data was also used. Multispectral data can be utilized to accurately assess coverage at the square inch level. From the normalized difference vegetation index (NDVI), an estimate of fractional vegetation coverage (FVC) was calculated, and multispectral data and maximum likelihood classifiers were also used to map soil features. Multispectral sensors are also beneficial because they can reveal small changes in vegetative cover over time.
When it comes to using drones, the data can become overwhelming, but it can be simplified with AI and machine learning. For this site, CDM Smith developed a custom above ground biomass machine learning model to track the growth of the salt marsh grass. The parameters this model used were elevation data and multispectral indices, such as a normalized difference vegetation index (NDVI), a normalized difference red edge (NDRE), and normalized difference water index (NDWI). See a visualization of the model below:
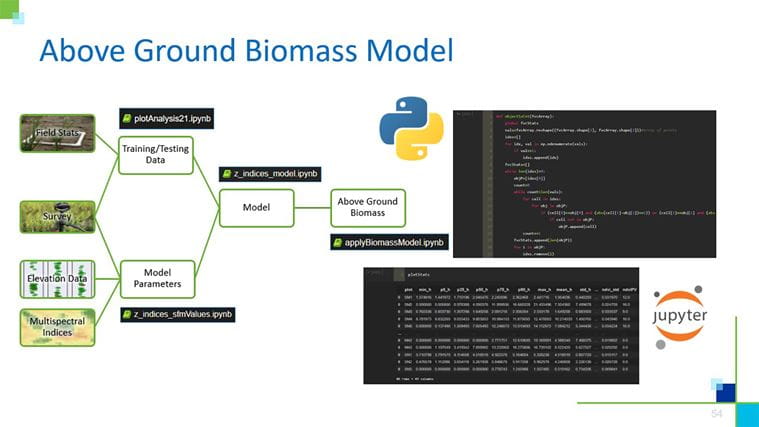
The Tidal Salt Marsh Restoration project demonstrates how drones can provide robust data sets, that can be analyzed by regulators and stakeholders to make decisions best fit for a site.
Vero Beach, Florida
The second case discussed explained how CDM Smith used drone data and machine learning to monitor blue carbon in Vero Beach, Florida. Red mangroves, a native species, has a different spectral signature than Australian pines, an invasive species. Using this multispectral data, CDM Smith created a custom machine learning model with over 90 percent accuracy in identifying the two species. What can be taken away from this case study is that drones and machine learning can fill gaps between traditional field transects and plane-based mapping, as traditional field transects are time consuming and provide smaller amounts of information, and planes provide courser resolution imaging compared to drones. Drones can provide both high resolution imaging and are more efficient than traditional field transects.
Hillsborough County, Florida
To make sense of this complex environmental site, CDM Smith used multispectral sensors and machine learning models to map invasive and native species, similarly to the previous case. 10-band data can produce informative maps for particular species, and through CDM Smith’s utilization of a custom machine learning model, the overall accuracy for fifteen species at the site was 80 percent accurate. Using machine learning models, CDM Smith was able to assess percentage of invasive species at a high resolution. An advantage of invasive species mapping through machine learning models is how these models can identify founding populations of invasive species which in turn facilitates eradication programs. Furthermore, machine learning models like this can be reapplied to completely new sites as a screening tool. Overall, model outputs like invasive species maps help land managers make decisions to restore and preserve habitats.
Summary of Drone and Machine Learning Capabilities at these Sites
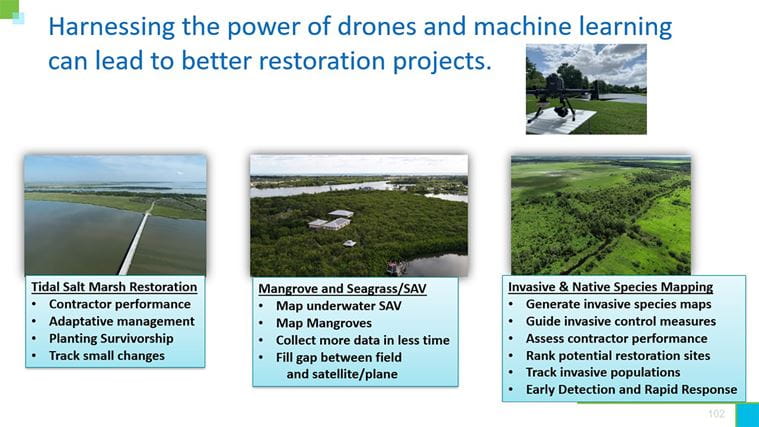
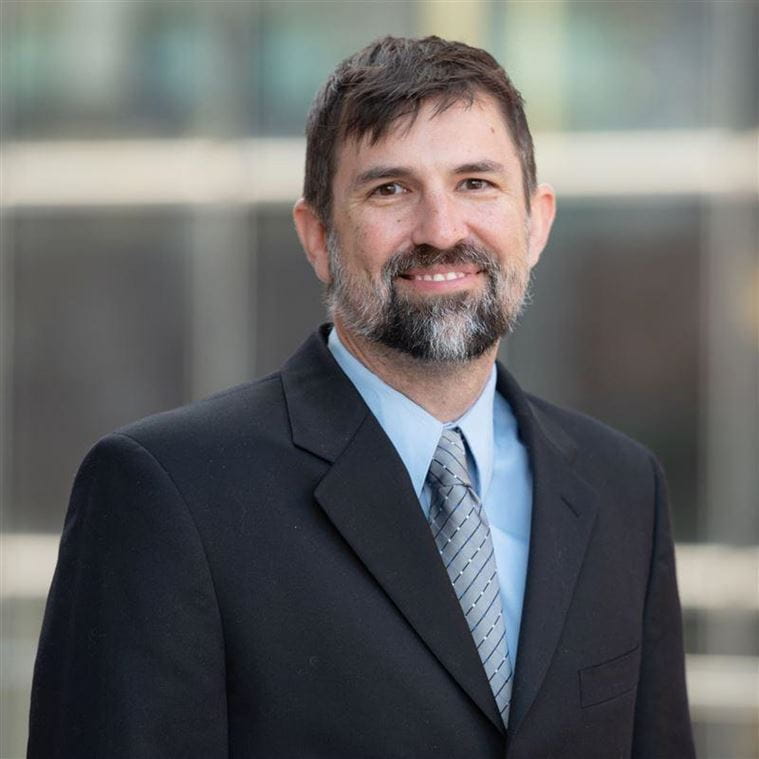
Drone technology both maximizes our time in the field and allows us to collect more meaningful and useful information
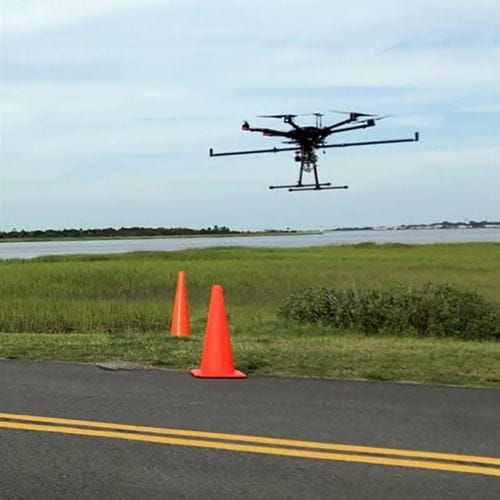